class: left, bottom background-image: url("images/contour.png") background-position: right background-size: auto # Character & String Data ### Environmental Data Literacy <p> </p> <p> </p> <img src="images/logo1.svg" width="400px"> --- class: sectionTitle # Textual Represenations --- # String & Character Data One of the most fundamental kinds of data we work with is textual data. - Population Names - Genetic Sequences - Parsing Raw Files - Automated Lexicographic Analyses --- # Character Data Types In `R`, string and character data is represented as a sequence of objects *enclosed* within either single or double quotes. ```r "Dyer" ``` ``` ## [1] "Dyer" ``` ```r 'Rodney' ``` ``` ## [1] "Rodney" ``` -- Notice that you .red[must] use the same quote character to start and finish the data within the sequence. --- # First Gotcha π₯ RStudio ties to match quotes (e.g., when you open as string with a quote glyph, it automatically puts in the matching closing one for you). However if this fails, RStudio will continue to accept input (forever) until you finish with the closed quote character. -- ``` > "Bob + + + + help() + + + + o crap, what is happening? + ``` The .red[dead] giveaway is the the plus sign that is automatically put into the next line on the console. --- # Creating String Variables In `R`, data is represented in *variables*, which is pretty handy so we do not need to type in a ton data each time we want to reference it. ```r string1 <- "Data Literacy is my favorite class!" ``` -- Variable names should: - Be unique - Start with a character - Contain letters, numbers, and some symbols (e.g., `_`, `.`) - Unless transient, it should .red[describe the data it is representing] --- # The Assignment Operator `<-` (or equally valid as `->`) To assign values to a variable, we use either the left or right *assignment operator* consisting of a dash and a less than or greater than symbol. Yes it is two symbols combined together (and in fact can point in either direction and have one or two of the *-than signs) ```r x <- "Bob" x ``` ``` ## [1] "Bob" ``` ```r "Alice" -> y y ``` ``` ## [1] "Alice" ``` -- You can also use two directional signs to give emphasis. ```r "Kingsley" ->> NedPlimptonsOtherName NedPlimptonsOtherName ``` ``` ## [1] "Kingsley" ``` --- # Why not the =? The grammar of `R` (neΓ© `S`) was defined using assignment as a .red[directional] operator to help *readability*. The equals sign is non-directional. -- In some cases you could use `=` in place of `<-` but it will not work properly .red[all the time]. For example if you look at `?Syntax`, the *Order of Operations* for assignment is different, with the equals sign below that of either `->` and `<-` ``` -> ->> Rightwards Assignment <- <<- Leftwards Assignment = Assignment (Right to Left) ``` This means that as things become more complex (which we will soon jump into), you can get some grammatical and logical errors if you .red[incorrectly insist] on using `=` instead of either `<-` or `->` for assignment. --- # Equality Operator While we are here with the equals sign, let's jump onto the issue of equality (e.g., testing to see if the data pointed by two or more variables are equal). This is done by using .red[two equals signs].<sup>1</sup> ```r x == y ``` ``` ## [1] FALSE ``` -- For inequality, we use this. ```r y != x ``` ``` ## [1] TRUE ``` .footnote[<sup>1</sup>This is another reason why we do not use `=` for assignment, it is just too easy to have a typo when you were intending to ask `y = 42` (assignment) vs `y == 42` (test of equality).] --- # Operations On Variables .pull-left[ The purpose of `functions` are to *encapsulate* a bunch of code. ```r # Make some random data N <- 100 x <- rnorm( N ) y <- rnorm( N ) # Plot it in a scatter plot plot( x, y ) ``` Consider how many things need to happen to make a simple scatter plot between two sets of random numbers. The function `rnorm()` provides a set of random numbers drawn from a normal distribution with mean `\(0\)` and variance `\(1\)`. ] .pull-right[ 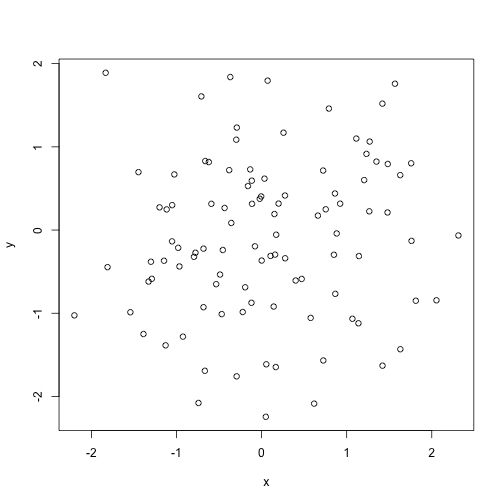<!-- --> ] --- # Operations on Variable We can apply `functions` to variables (or to nothing) that do operations for us. The form a function is ``` functionName( varible1, variable2, ...) ``` -- The name of the function come first followed by zero or more variables **within** parenthesesβeach separated by a comma.<sup>1</sup> - Functions provide a nice way for us to **encapsulate** code that does stuff for us (often over and over to make our lives enjoyable). - To find out about the function, you can use the *Help* pane in `RStudio` or in the console type `?functionName` and it will show the help file for you. - β οΈ Please make sure there is no space between the end of the name of the function and the opening parenthesis! .footnote[<sup>1</sup>The ellipsis is for additional stuff to be added if present and is used to allow the user to pass additional information to *downstream* operations.] --- # Investigating Contents of String Variables π€ There are times when we need to figure out properties of characters within a string object. ```r NedPlimptonsOtherName ``` ``` ## [1] "Kingsley" ``` ```r # Number of characters in the data pointed to by the variable NedPlimptonsOtherName nchar( NedPlimptonsOtherName ) ``` ``` ## [1] 8 ``` .footnote[Programmers are .red[lazy] and whenever we can do something that allows us to type fewer characters, we will do it. This reduces the opportunity for us screwing up and introducing errors into our code.] --- class: reveal # Special 'Escaped' Characters There are some keys on your keyboard that have some special meaning that cannot be represented by a single *glyph* in a text file. There are various ways to display these. RStudio + Keyboard Input - Tab character (ascii keyboard input): β₯ - New Line (Carriage Return + Line Feed; ascii keyboard input as well): β² - ASCII Escaped Characters (backslash escaping of special characters): Β―\\\_(γ)\_/Β― `R` Interpreted Input - Greek and Latin Symbols (keyboard combinations or unicode): Β΅ - Emoji (yes, there is a library to insert the poop emoji): π© --- # Quoting Quotes There are times that you need to actually use a quote character in our output. To do this, we use the backslash escape. ```r x <- "\"" x ``` ``` ## [1] "\"" ``` -- By default, `R` will show you the escaped version as normal `R` code. However, if you want to write it out as it would be if we saved it to a file, you can use the `writeLines()` function (the same applies for [unicode](https://unicode-table.com/en/) stuff). ```r writeLines(x) ``` ``` ## " ``` ```r writeLines("\u2665") ``` ``` ## β₯ ``` --- # Concatenating String Objects - Your First Function π The `c()` function is the first and shortest of built-in functions. The purpose of this is to take more than one instance of a variable and concatenate them as a *vector*. ```r names <- c( "Ned", "Plimpton") names ``` ``` ## [1] "Ned" "Plimpton" ``` -- To access them individually, we use square brackets `[` and `]` and a numerical index (beware these start counting at 1 you nascent python users). ```r names[2] ``` ``` ## [1] "Plimpton" ``` ```r names[3] ``` ``` ## [1] NA ``` --- # Increasing Concatenation You can subsequently add more items to a vector by assignemnt to existing entries ```r names[1] <- "Kingsley" names ``` ``` ## [1] "Kingsley" "Plimpton" ``` -- Or to ones that are beyond the current range (a generally bad habit thought). ```r names[4] <- "Zissou" names ``` ``` ## [1] "Kingsley" "Plimpton" NA "Zissou" ``` β οΈ There is a `NA` (which is missing data) in the 3<sup><u>rd</u></sup> position. --- # Performance Recommendation π If you are going to work with large vectors, it is in your best interest to not incrementally concatenate data. ``` x <- "Bob" x <- c( x, "Alice" ) x <- c( x, "Roger" ) ... ``` -- The best way is to preallocate your data vector to the size you need it and then fill it in. Depending upon the kind of variable you are using, it will put in the default `null` value for each entry. Then as you need it, fill it in. ```r x <- character( 12 ) x ``` ``` ## [1] "" "" "" "" "" "" "" "" "" "" "" "" ``` --- # Lengths of Things π We've already seen the `nchar()` function that gives the number of characters is a variable. ```r names ``` ``` ## [1] "Kingsley" "Plimpton" NA "Zissou" ``` ```r nchar( names ) ``` ``` ## [1] 8 8 NA 6 ``` -- But if we want the number of entries in the vector itself, we use `length()`. ```r length( names ) ``` ``` ## [1] 4 ``` .footnote[We will see shortly when we jump into using `stringr` that we can disambiguate these things a bit.] --- # Built-In String Objects There are some built-in variables that provide string objects for you. ```r LETTERS ``` ``` ## [1] "A" "B" "C" "D" "E" "F" "G" "H" "I" "J" "K" "L" "M" "N" "O" "P" "Q" "R" "S" ## [20] "T" "U" "V" "W" "X" "Y" "Z" ``` The `LETTERS` object in `R` is a *variable* that contains data (in this case a sequence of letters). --- class: sectionTitle # Packages ### Extending Built-In Functionality with Someone Elses Efforts π --- # Packages .pull-left[ Packages are collections of code that can include: - Functions - Help files - Example data - Images - Documentation - Vignettes ] -- .pull-right[ You can install packages by typing the command `install.packages()` into the console. Let's install a package that allows you to insert emoji's (why not) and another that will do some more specific string operations because we are trying to be serious here. The first packages is installed from Hadley's [Github](https://github.com/hadley/emo) repository and the second one is from the global [CRAN](https://cran.r-project.org) repository. ```r install.packages("stringr") remotes::install_github("hadley/emo") ``` ] --- # Loading Packages Now that they are installed on your computer, you need to tell `R` when you want to use them. By default, they are not loaded into memory because there can literally be too many packages on your machine for the limited amount of RAM you have. I currently have 418 different packages installed onto my laptop as I'm typing this. To load them in, we use `library()` ```r library(stringr) ``` Now all the functions in the `stringr` package are in memory. --- # While We Are At It While we are here, let's just do this once and get it over with. Instead of loading in the individuals parts of tidyverse, let's just get in the habit of loading it all in at the beginning. So to install all of tidyverse, ```r install.packages("tidyverse") ``` Then when we start we can use: ```r library( tidyverse ) ``` ``` ## ββ Attaching packages βββββββββββββββββββββββββββββββββββββββ tidyverse 1.3.1 ββ ``` ``` ## β ggplot2 3.3.5 β purrr 0.3.4 ## β tibble 3.1.4 β dplyr 1.0.7 ## β tidyr 1.1.3 β forcats 0.5.1 ## β readr 2.0.1 ``` ``` ## ββ Conflicts ββββββββββββββββββββββββββββββββββββββββββ tidyverse_conflicts() ββ ## x dplyr::filter() masks stats::filter() ## x dplyr::lag() masks stats::lag() ``` To get everything in at the beginning so we don't have to worry about it later. --- # Not Loading but Accessing There are times (and we will be very aware of this when we get to working with spatial data) when the process of loading in a bunch of functions from a package can cause some problems. This is especially an issue when the name of functions in two or more libraries are the same. How is `R` supposed to know which one to use? -- ### Package Namespaces What `R` has done is to create package namespaces that allow you to grab a function from a package without loading the whole package into memory. To do this, we use the full package name and the function name connected by two colons (`::`). ``` packageName::function() ``` -- For the Emoji library (`emo`), this was used exclusively since it was thought that you'd never actually want to load them all into memory. ```r emo::ji("poop") ``` ``` ## π© ``` --- class: sectionTitle # The `stringr` Library --- # Exploring Package Functions Each package must have a large amount of help files and documentation before CRAN allows it to be put on the global servers. You can find it using the built-in help in RStudio or by using ```r ?stringr ``` or ```r help.search("stringr") ``` --- # The Purpose of `stringr` The `stringr` package is part of a constellation of packages known as `tidyverse` that focues on string operations. .pull-left[ > The tidyverse is an *opinionated* collection of R packages designed for data science. All packages share an underlying design philosophy, grammar, and data structures. ] .pull-right[ <img src="https://d33wubrfki0l68.cloudfront.net/b88ef926a004b0fce72b2526b0b5c4413666a4cb/ad0a8/images/cover.png" height=300> ] Some of the functions in `stringr` replicate built-in functions but since we are going to use tidyverse explicitly in this class, we will skip over themβa problem with the built-in stuff is *inconsistency*, which is why tidyverse was created. --- # Common Prefix All of the functions in `stringr` start with `str_` as a prefix. This is to help prevent *namespace collisions* as well as to make sure you are entirely confident that the functions you are using are the ones you think you are using. --- # Length of String Objects Since `length` is such a common characteristic, `str_length` is what `nchar` does but in a more consistent taxonomic context. ```r str_length( names ) ``` ``` ## [1] 8 8 NA 6 ``` -- For the length of the vector, we still use `length()` as it is asking for the number of elements in the vector, **not** the length of the string elements within it. ```r length( names ) ``` ``` ## [1] 4 ``` .footnote[ See, this is much better than remembering `nchar` vs `lenght`.] --- # Concatenating Strings By default, concatenation is direct. ```r str_c("Rodney","Dyer") ``` ``` ## [1] "RodneyDyer" ``` But there are times when we need to insert some character into the concatenation so it works ```r str_c( "Rodney", "Dyer", sep = " ") ``` ``` ## [1] "Rodney Dyer" ``` --- # Dropping Missing Values Missing data is a fact of life. In fact, "If you do not have missing data somewhere, you are not trying hard enough..." said my advisor once. In `R`, all missing data is encoded as `NA` (which is actually a data type) ```r names ``` ``` ## [1] "Kingsley" "Plimpton" NA "Zissou" ``` -- It can be identified. ```r is.na( names ) ``` ``` ## [1] FALSE FALSE TRUE FALSE ``` -- And replaced ```r str_replace_na(names, replacement = "Ned") ``` ``` ## [1] "Kingsley" "Plimpton" "Ned" "Zissou" ``` --- # Vectorization Since .red[a lot] of the work we do in `R` is on vectors of data, the functions must also be *vectorized*. ```r myClasses <- str_c("ENVS", c(521, 543, 601, 602), sep="-" ) myClasses ``` ``` ## [1] "ENVS-521" "ENVS-543" "ENVS-601" "ENVS-602" ``` -- Two Items of Interest here: 1. Notice how the prefix `ENVS` was merged into with each element of the class vector and the `sep` indicates the separator between them. 1. The numbers in the vector were converted (coerced) into character values directly. Coercion is a one-directional monster and a string represenation of another data type is one of the lowest precidents. --- # Collapsing = `\(\frac{1}{Vectorization}\)` You can go the other way in this and take a vector of results and collapse them back into a single string. ```r str_c( myClasses, collapse=", ") ``` ``` ## [1] "ENVS-521, ENVS-543, ENVS-601, ENVS-602" ``` --- # Grabbing Subsets Components within elements can be extracted using the numerical index of the first and last component of interest (n.b., both indices are inclusive). ```r str_sub(myClasses, start = 6, end = 8) ``` ``` ## [1] "521" "543" "601" "602" ``` -- Counting backwards too (notice I also can drop the names of the non-data variables too - but **must** be given in the order displayed in the help file!). ```r str_sub(myClasses, -3, -1) ``` ``` ## [1] "521" "543" "601" "602" ``` .red[Look mom, I'm going backwards π€ͺ !] --- class: sectionTitle # Regular Expressions ## Searching with Wildcards --- # Regular Expressions This could be a whole .red[frickin] class in itself. I'm going to keep it very simple here so you have enough knowledge to get in trouble. > Regular Expression is a sequence of characters that defines a search pattern. These are **very terse** descriptions of textual patterns. -- ```r names[3] <- "Ted Knight" names ``` ``` ## [1] "Kingsley" "Plimpton" "Ted Knight" "Zissou" ``` --- # Visualizing Matches for Learning The function `str_view()` is designed to **only** show you where matches are made to help you learn regex, we **never** actually use it in practice. Visualizing matches for the letter *i* ```r str_view( names, "i") ```
--- # Positional Matches Matching based upon position can be used with the addition of a special character. Here the `$` indicates that it is at the .red[very end] of the string. ```r str_view( names, "n$") ```
--- # Positional Matches And the `^` character marks .red[the beginning] of a string. ```r str_view( names, "^K") ```
Notice how it *does not* match the 'K' in Knight. --- # Character Classes We can also match kinds of characters such as a number, whose shortcut is `\d`. However, to have it match as a character class, we need to be careful of the `\` character. There are some special characters such as `\t` (tab), `\r` (carrage return), and `\n` newline that are shorthand ways of indicating these non-printing entities on your keyboard. As such, a `\` is treated .red[speically] to indicate that the next glyph will indicate a special character. But if we try to use it like this: ```r str_view( myClasses, "\d" ) ``` .red[```# Error: '\d' is an unrecognized escape in character string starting ""\d"```] Which is an error. --- # Character Classes This is because the digit indication for a regular expression includes a `\` not as a "escape character" but as part of it directly! So, we need to escape it as well (confused yet? stick with me, it will get better). So to match the first generic number in each of the entries, we would use ```r str_view( myClasses, "\\d" ) ```
--- # Number of Character Classes You can match more than one of the characters. ```r str_view(myClasses, "\\d\\d") ```
--- # Matching "other" The `"."` character indicates another glyph (of any type) ```r str_view( myClasses, "\\d.+") ```
--- # Finding Words We can also match on 'words' or 'whitespace'. ```r text <- "It was the best of times, it was the worst of times, it was the age of wisdom, it was the age of foolishness, it was the epoch of belief, it was the epoch of incredulity, it was the season of light, it was the season of darkness, it was the spring of hope, it was the winter of despair." ``` This grabs the first word (grabbed by the `\\w` that is immediately followed by a comma.) ```r str_view( text, "\\w\\,") ```
```r str_view( text, "\\w\\s(belief)") ```
--- # Numbers of matches We can match things by the number of occurrences. Consider the following searches for the lowercase letter `o`. - `o*` Finding zero or more times - `o+` Finding one or more times. - `o?` Either 0 or 1 times ```r str_view( text, "o+") ```
--- # Finding `N` items. ```r year_1888 <- "MDCCCLXXXVIII" str_view( year_1888, "X{3}") ```
--- # Variable Numbers of Matches How about finding where the string contains either 2 consecutive lowercase 'o' values? ```r str_view( text, "o{2,3}" ) ```
--- # A Greedy Algorithm Given the opportunity, it will identify the location of the longest match. ```r str_view( year_1888, "C{1,4}") ```
--- # Locating the Position of Elements ```r str_locate( text, "epoch") ``` ``` ## start end ## [1,] 122 126 ``` --- # Locating Every Instance of an Element Finding *all* the start and ending positions for a particular sequence. ```r str_locate_all( text, "it was the") ``` ``` ## [[1]] ## start end ## [1,] 27 36 ## [2,] 54 63 ## [3,] 80 89 ## [4,] 111 120 ## [5,] 139 148 ## [6,] 172 181 ## [7,] 200 209 ## [8,] 231 240 ## [9,] 258 267 ``` -- From this `str_sub()` can be used to extract elements within (or between) identified occurances. Here is an example of the ```r str_sub( text, 80, 110) ``` ``` ## [1] "it was the age of foolishness, " ``` --- # Extracting Elements We can do a similar thing to pull out the components. ```r str_extract( text, "epoch") ``` ``` ## [1] "epoch" ``` ```r str_extract_all( text, "it was the") ``` ``` ## [[1]] ## [1] "it was the" "it was the" "it was the" "it was the" "it was the" ## [6] "it was the" "it was the" "it was the" "it was the" ``` --- # Some More Options It will be more exciting if we could use `str_extract()` in a way that allows us to capture more than just **exactly** what we asked for it to find. Item | Definition -----|----------------------------- `^` | The start of a string match `$` | The end of a string match `(` | The start of a capture group `)` | The end of a capture group --- # More Exciting, no? So, if we want to find the .red[word] that occurs right before the comma in the text, we create a *capture group* representing one or more word elements (`\w`) followed by a comma. ```r str_extract_all( text, "(\\w*)," ) ``` ``` ## [[1]] ## [1] "times," "times," "wisdom," "foolishness," "belief," ## [6] "incredulity," "light," "darkness," "hope," ``` -- Simplifying the result a bit. ```r str_extract_all( text, "(\\w*),", simplify = TRUE) ``` ``` ## [,1] [,2] [,3] [,4] [,5] [,6] ## [1,] "times," "times," "wisdom," "foolishness," "belief," "incredulity," ## [,7] [,8] [,9] ## [1,] "light," "darkness," "hope," ``` --- # Matching Sequences We can define sequences by enclosing them into parentheses. So to search for all entries that have a `6` and a `0` right next to each other, we could. ```r str_view( myClasses, "(60)") ```
```r str_view( myClasses, "ENVS-(60)") ```
--- # Optional Characters - This OR That This may be convenient when there are a few different kinds of spellings. ```r str_view( c("gray", "grey"), "r(a|e)y") ```
--- # Numbers of Matches in a Sequence Let's look at a larger set of words and do some actual detection where we are not intersted in showing the results (using `str_view()` but working with the answers themselves). Let's use the built-in `words` data, which has ... words ```r head( words ) ``` ``` ## [1] "a" "able" "about" "absolute" "accept" "account" ``` ```r tail( words ) ``` ``` ## [1] "year" "yes" "yesterday" "yet" "you" "young" ``` ```r length( words ) ``` ``` ## [1] 980 ``` --- # Counts of Items Simply finding something may be good for determining if something exists. However, we may want to count how many occurrences of something are in the text string. Here is an example where we use `str_detect()` to return `TRUE/FALSE` for matching the pattern and then count how many `TRUE` results there are. ```r startingWithR <- str_detect( words, "^r") sum( startingWithR ) ``` ``` ## [1] 46 ``` -- Could combine the two (looking at words ending in `r` this time) as: ```r sum( str_detect( words, "r$") ) ``` ``` ## [1] 77 ``` --- # Negation (the opposite of) Fraction of words starting with a vowel in vowel ```r mean( str_detect( words, "^[aeiou]") ) ``` ``` ## [1] 0.1785714 ``` -- vs. the fraction that **DO NOT** start with a vowel ```r mean( !str_detect( words, "^[aeiou]") ) ``` ``` ## [1] 0.8214286 ``` (n.b., these two numbers *better* sum to 1.0!) --- # Selecting Elements The function `str_detect()` is commonly used to find elements in an array that match an expression and then use this result as an index on the sequences to pull them out. ```r words[ str_detect( words, "r$") ] ``` ``` ## [1] "after" "air" "another" "answer" "appear" ## [6] "bar" "bear" "bother" "brother" "car" ## [11] "chair" "character" "clear" "colour" "confer" ## [16] "consider" "corner" "cover" "danger" "dear" ## [21] "dinner" "doctor" "door" "either" "enter" ## [26] "ever" "fair" "far" "father" "favour" ## [31] "floor" "for" "four" "further" "hair" ## [36] "hear" "hour" "however" "labour" "letter" ## [41] "major" "matter" "member" "minister" "mister" ## [46] "mother" "near" "never" "number" "offer" ## [51] "or" "order" "other" "over" "pair" ## [56] "paper" "particular" "per" "poor" "power" ## [61] "proper" "quarter" "rather" "refer" "remember" ## [66] "similar" "sir" "sister" "summer" "together" ## [71] "under" "war" "water" "wear" "whether" ## [76] "wonder" "year" ``` --- ```r str_detect( words, "r$") ``` ``` ## [1] FALSE FALSE FALSE FALSE FALSE FALSE FALSE FALSE FALSE FALSE FALSE FALSE ## [13] FALSE FALSE FALSE FALSE FALSE TRUE FALSE FALSE FALSE FALSE FALSE FALSE ## [25] FALSE TRUE FALSE FALSE FALSE FALSE FALSE FALSE FALSE FALSE FALSE FALSE ## [37] FALSE FALSE TRUE TRUE FALSE FALSE FALSE TRUE FALSE FALSE FALSE FALSE ## [49] FALSE FALSE FALSE FALSE FALSE FALSE FALSE FALSE FALSE FALSE FALSE FALSE ## [61] FALSE FALSE FALSE FALSE FALSE FALSE FALSE FALSE FALSE FALSE FALSE FALSE ## [73] TRUE FALSE FALSE FALSE TRUE FALSE FALSE FALSE FALSE FALSE FALSE FALSE ## [85] FALSE FALSE FALSE FALSE FALSE FALSE FALSE FALSE FALSE FALSE FALSE FALSE ## [97] FALSE FALSE FALSE FALSE FALSE FALSE FALSE FALSE TRUE FALSE FALSE FALSE ## [109] FALSE FALSE FALSE FALSE FALSE FALSE TRUE FALSE FALSE FALSE FALSE FALSE ## [121] FALSE FALSE FALSE FALSE FALSE FALSE TRUE FALSE FALSE FALSE FALSE FALSE ## [133] FALSE FALSE FALSE FALSE FALSE TRUE FALSE FALSE FALSE FALSE TRUE FALSE ## [145] FALSE FALSE FALSE FALSE FALSE FALSE FALSE FALSE FALSE FALSE FALSE FALSE ## [157] TRUE FALSE FALSE FALSE FALSE FALSE FALSE FALSE FALSE FALSE FALSE FALSE ## [169] TRUE FALSE FALSE FALSE FALSE FALSE FALSE FALSE FALSE FALSE FALSE FALSE ## [181] FALSE TRUE TRUE FALSE FALSE FALSE FALSE FALSE FALSE FALSE FALSE TRUE ## [193] FALSE FALSE FALSE FALSE FALSE FALSE FALSE FALSE FALSE FALSE TRUE FALSE ## [205] FALSE FALSE FALSE FALSE FALSE TRUE FALSE FALSE FALSE FALSE TRUE FALSE ## [217] FALSE FALSE FALSE FALSE FALSE FALSE FALSE FALSE FALSE FALSE FALSE FALSE ## [229] FALSE FALSE TRUE FALSE FALSE FALSE FALSE FALSE TRUE FALSE FALSE TRUE ## [241] FALSE FALSE FALSE FALSE FALSE FALSE FALSE FALSE FALSE FALSE FALSE FALSE ## [253] FALSE FALSE FALSE FALSE FALSE FALSE FALSE FALSE FALSE TRUE FALSE FALSE ## [265] FALSE FALSE FALSE FALSE FALSE FALSE FALSE FALSE FALSE TRUE FALSE FALSE ## [277] FALSE FALSE FALSE FALSE TRUE FALSE FALSE FALSE FALSE FALSE FALSE FALSE ## [289] FALSE FALSE FALSE FALSE FALSE FALSE FALSE FALSE FALSE FALSE TRUE FALSE ## [301] FALSE TRUE FALSE FALSE TRUE TRUE FALSE FALSE FALSE FALSE FALSE FALSE ## [313] FALSE FALSE FALSE FALSE FALSE FALSE FALSE FALSE FALSE FALSE FALSE FALSE ## [325] FALSE FALSE TRUE FALSE FALSE FALSE FALSE TRUE FALSE FALSE FALSE FALSE ## [337] FALSE TRUE FALSE FALSE FALSE FALSE FALSE FALSE FALSE FALSE FALSE FALSE ## [349] TRUE FALSE FALSE FALSE FALSE FALSE FALSE FALSE FALSE FALSE FALSE FALSE ## [361] FALSE FALSE FALSE FALSE FALSE FALSE FALSE FALSE FALSE FALSE FALSE FALSE ## [373] FALSE TRUE FALSE FALSE FALSE FALSE FALSE FALSE FALSE FALSE FALSE FALSE ## [385] FALSE FALSE TRUE FALSE FALSE FALSE FALSE FALSE FALSE FALSE FALSE FALSE ## [397] FALSE FALSE FALSE FALSE FALSE FALSE FALSE FALSE TRUE FALSE FALSE TRUE ## [409] FALSE FALSE FALSE FALSE FALSE FALSE FALSE FALSE FALSE FALSE FALSE FALSE ## [421] FALSE FALSE FALSE FALSE FALSE FALSE FALSE FALSE FALSE FALSE FALSE FALSE ## [433] FALSE FALSE FALSE FALSE FALSE FALSE FALSE FALSE FALSE FALSE FALSE FALSE ## [445] FALSE FALSE FALSE FALSE FALSE FALSE FALSE TRUE FALSE FALSE FALSE FALSE ## [457] FALSE FALSE FALSE FALSE FALSE FALSE FALSE FALSE FALSE FALSE FALSE FALSE ## [469] FALSE TRUE FALSE FALSE FALSE FALSE FALSE FALSE FALSE FALSE FALSE FALSE ## [481] FALSE FALSE FALSE FALSE FALSE FALSE FALSE FALSE FALSE FALSE FALSE FALSE ## [493] FALSE FALSE FALSE FALSE FALSE FALSE TRUE FALSE FALSE FALSE FALSE FALSE ## [505] FALSE FALSE FALSE TRUE FALSE FALSE FALSE FALSE FALSE FALSE TRUE FALSE ## [517] FALSE FALSE FALSE FALSE FALSE FALSE TRUE FALSE FALSE FALSE TRUE FALSE ## [529] FALSE FALSE FALSE FALSE FALSE FALSE TRUE FALSE FALSE FALSE FALSE FALSE ## [541] FALSE FALSE FALSE FALSE TRUE FALSE FALSE TRUE FALSE FALSE FALSE FALSE ## [553] FALSE FALSE FALSE FALSE FALSE FALSE FALSE FALSE FALSE FALSE FALSE TRUE ## [565] FALSE FALSE FALSE FALSE FALSE TRUE FALSE FALSE FALSE FALSE FALSE FALSE ## [577] FALSE FALSE FALSE FALSE FALSE FALSE TRUE TRUE FALSE FALSE TRUE FALSE ## [589] FALSE FALSE TRUE FALSE FALSE FALSE FALSE TRUE TRUE FALSE FALSE FALSE ## [601] FALSE FALSE TRUE FALSE FALSE FALSE FALSE FALSE FALSE FALSE TRUE FALSE ## [613] FALSE FALSE FALSE FALSE FALSE FALSE FALSE FALSE FALSE FALSE FALSE FALSE ## [625] FALSE FALSE FALSE FALSE FALSE TRUE FALSE FALSE FALSE FALSE FALSE TRUE ## [637] FALSE FALSE FALSE FALSE FALSE FALSE FALSE FALSE FALSE FALSE FALSE FALSE ## [649] FALSE FALSE FALSE FALSE FALSE FALSE FALSE TRUE FALSE FALSE FALSE FALSE ## [661] FALSE FALSE FALSE FALSE FALSE TRUE FALSE FALSE FALSE FALSE FALSE FALSE ## [673] FALSE FALSE FALSE FALSE TRUE FALSE FALSE FALSE FALSE FALSE FALSE FALSE ## [685] FALSE FALSE FALSE FALSE FALSE FALSE FALSE TRUE FALSE FALSE FALSE TRUE ## [697] FALSE FALSE FALSE FALSE FALSE FALSE FALSE FALSE FALSE FALSE FALSE FALSE ## [709] FALSE FALSE FALSE FALSE FALSE FALSE FALSE FALSE FALSE FALSE FALSE FALSE ## [721] FALSE FALSE FALSE FALSE FALSE FALSE FALSE FALSE FALSE FALSE FALSE FALSE ## [733] FALSE FALSE FALSE FALSE FALSE FALSE FALSE FALSE FALSE FALSE FALSE FALSE ## [745] FALSE FALSE FALSE FALSE FALSE FALSE FALSE FALSE FALSE FALSE FALSE FALSE ## [757] FALSE FALSE FALSE FALSE FALSE TRUE FALSE FALSE FALSE FALSE TRUE TRUE ## [769] FALSE FALSE FALSE FALSE FALSE FALSE FALSE FALSE FALSE FALSE FALSE FALSE ## [781] FALSE FALSE FALSE FALSE FALSE FALSE FALSE FALSE FALSE FALSE FALSE FALSE ## [793] FALSE FALSE FALSE FALSE FALSE FALSE FALSE FALSE FALSE FALSE FALSE FALSE ## [805] FALSE FALSE FALSE FALSE FALSE FALSE FALSE FALSE FALSE FALSE FALSE FALSE ## [817] FALSE FALSE FALSE FALSE FALSE FALSE FALSE FALSE FALSE FALSE TRUE FALSE ## [829] FALSE FALSE FALSE FALSE FALSE FALSE FALSE FALSE FALSE FALSE FALSE FALSE ## [841] FALSE FALSE FALSE FALSE FALSE FALSE FALSE FALSE FALSE FALSE FALSE FALSE ## [853] FALSE FALSE FALSE FALSE FALSE FALSE FALSE FALSE FALSE FALSE FALSE FALSE ## [865] FALSE FALSE FALSE FALSE FALSE FALSE FALSE FALSE FALSE FALSE FALSE TRUE ## [877] FALSE FALSE FALSE FALSE FALSE FALSE FALSE FALSE FALSE FALSE FALSE FALSE ## [889] FALSE FALSE FALSE FALSE FALSE FALSE FALSE FALSE FALSE FALSE FALSE FALSE ## [901] FALSE TRUE FALSE FALSE FALSE FALSE FALSE FALSE FALSE FALSE FALSE FALSE ## [913] FALSE FALSE FALSE FALSE FALSE FALSE FALSE FALSE FALSE FALSE FALSE FALSE ## [925] FALSE FALSE TRUE FALSE FALSE FALSE FALSE TRUE FALSE FALSE TRUE FALSE ## [937] FALSE FALSE FALSE FALSE FALSE FALSE FALSE FALSE FALSE TRUE FALSE FALSE ## [949] FALSE FALSE FALSE FALSE FALSE FALSE FALSE FALSE FALSE FALSE FALSE FALSE ## [961] FALSE FALSE FALSE TRUE FALSE FALSE FALSE FALSE FALSE FALSE FALSE FALSE ## [973] FALSE FALSE TRUE FALSE FALSE FALSE FALSE FALSE ``` --- # Splitting Strings It is common for us to try to split strings into sections. It could be for files we get from a computer (relative to the starting: ```r geoTIFFS <- list.files(path = "/Users/rodney/Documents/github/classes/ENVS-Lectures", recursive = TRUE, pattern = "tif" ) geoTIFFS ``` ``` ## [1] "data/alt_22.tif" ## [2] "data/Annual_Precip_22.tif" ## [3] "data/DEM_5m.tif" ## [4] "data/Maximum_Precip.tif" ## [5] "data/Maximum_Temp_22.tif" ## [6] "data/Mean_Temp_22.tif" ## [7] "data/Minimum_Precip_22.tif" ## [8] "data/Minimum_Temp_22.tif" ## [9] "data/NLCD_2011_Land_Cover_L48_20190424_qn2B1f8ganicJNKnJN0e.tiff" ## [10] "data/NLCD_2013_Land_Cover_L48_20190424_qn2B1f8ganicJNKnJN0e.tiff" ## [11] "data/NLCD_2016_Land_Cover_L48_20190424_qn2B1f8ganicJNKnJN0e.tiff" ## [12] "docs/r_language/environment/data/alt_22.tif" ## [13] "lectures/r_language/environment/data/alt_22.tif" ``` --- # Files & File Paths ```r file <- geoTIFFS[13] file ``` ``` ## [1] "lectures/r_language/environment/data/alt_22.tif" ``` -- It is so common to deal with file names (and so problematic because of the folder specifier is `\` but one platform incorrectly uses `/`) ```r basename(file) ``` ``` ## [1] "alt_22.tif" ``` ```r dirname( file ) ``` ``` ## [1] "lectures/r_language/environment/data" ``` --- .pull-left[ # `str_split()` Components When we split a string, it is returned as a `list` of objects. **Lists** - Lists are a kind of container - Allow different kinds of data - Indexed by **either** number or character key - Uses 2 [[ and 2 ]] for indexes. ] .pull-right[ ```r file_parts <- str_split( geoTIFFS, pattern="/") class( file_parts ) ``` ``` ## [1] "list" ``` ```r length( file_parts ) ``` ``` ## [1] 13 ``` ```r file_parts[1:5] ``` ``` ## [[1]] ## [1] "data" "alt_22.tif" ## ## [[2]] ## [1] "data" "Annual_Precip_22.tif" ## ## [[3]] ## [1] "data" "DEM_5m.tif" ## ## [[4]] ## [1] "data" "Maximum_Precip.tif" ## ## [[5]] ## [1] "data" "Maximum_Temp_22.tif" ``` ```r file_parts[[1]][2] ``` ``` ## [1] "alt_22.tif" ``` ] --- # From File Contents Or from the contents of an individual data file. This file can be local (as in `~/data/alt_22.tif` in the previous slide) or on a remote computer somewhere. Here is a data file that I use in teaching and is located in my github repository. It describes the 100 beer styles recognized for competition by the international Beer Judge Certification Program (BJCP and yes there is such an organization). ```r beerStyles <- readLines( "https://github.com/dyerlab/ENVS-Lectures/raw/master/data/Beer_Styles.csv") beerStyles[1:10] ``` ``` ## [1] "Styles,Yeast,ABV_Min,ABV_Max,IBU_Min,IBU_Max,SRM_Min,SRM_Max,OG_Min,OG_Max,FG_Min,FG_Max" ## [2] "American Light Lager,Lager,2.8,4.2,8,12,2,3,1.028,1.04,0.998,1.008" ## [3] "American Lager,Lager,4.2,5.3,8,18,2,4,1.04,1.05,1.004,1.01" ## [4] "Cream Ale,Ale,4.2,5.6,8,20,2.5,5,1.042,1.055,1.006,1.012" ## [5] "American Wheat Beer,Either,4,5.5,15,30,3,6,1.04,1.055,1.008,1.013" ## [6] "International Pale Lager,Either,4.6,6,18,25,2,6,1.042,1.05,1.008,1.012" ## [7] "International Amber Lager,Lager,4.6,6,8,25,7,14,1.042,1.055,1.008,1.014" ## [8] "International Dark Lager,Lager,4.2,6,8,20,14,22,1.044,1.056,1.008,1.012" ## [9] "Czech Pale Lager,Lager,3,4.1,20,35,3,6,1.028,1.044,1.008,1.014" ## [10] "Czech Premium Pale Lager,Lager,4.2,5.8,30,45,3.5,6,1.044,1.06,1.013,1.017" ``` --- ```r read_csv("https://github.com/dyerlab/ENVS-Lectures/raw/master/data/Beer_Styles.csv") ``` ``` ## Rows: 100 Columns: 12 ``` ``` ## ββ Column specification ββββββββββββββββββββββββββββββββββββββββββββββββββββββββ ## Delimiter: "," ## chr (2): Styles, Yeast ## dbl (10): ABV_Min, ABV_Max, IBU_Min, IBU_Max, SRM_Min, SRM_Max, OG_Min, OG_M... ``` ``` ## ## βΉ Use `spec()` to retrieve the full column specification for this data. ## βΉ Specify the column types or set `show_col_types = FALSE` to quiet this message. ``` ``` ## # A tibble: 100 Γ 12 ## Styles Yeast ABV_Min ABV_Max IBU_Min IBU_Max SRM_Min SRM_Max OG_Min OG_Max ## <chr> <chr> <dbl> <dbl> <dbl> <dbl> <dbl> <dbl> <dbl> <dbl> ## 1 Americanβ¦ Lager 2.8 4.2 8 12 2 3 1.03 1.04 ## 2 Americanβ¦ Lager 4.2 5.3 8 18 2 4 1.04 1.05 ## 3 Cream Ale Ale 4.2 5.6 8 20 2.5 5 1.04 1.06 ## 4 Americanβ¦ Eithβ¦ 4 5.5 15 30 3 6 1.04 1.06 ## 5 Internatβ¦ Eithβ¦ 4.6 6 18 25 2 6 1.04 1.05 ## 6 Internatβ¦ Lager 4.6 6 8 25 7 14 1.04 1.06 ## 7 Internatβ¦ Lager 4.2 6 8 20 14 22 1.04 1.06 ## 8 Czech Paβ¦ Lager 3 4.1 20 35 3 6 1.03 1.04 ## 9 Czech Prβ¦ Lager 4.2 5.8 30 45 3.5 6 1.04 1.06 ## 10 Czech Amβ¦ Lager 4.4 5.8 20 35 10 16 1.04 1.06 ## # β¦ with 90 more rows, and 2 more variables: FG_Min <dbl>, FG_Max <dbl> ``` .footnote[ `read_csv()` is in the `readr` library which is part of the `tidyverse.] --- class: middle background-image: url("images/contour.png") background-position: right background-size: auto .center[ # Questions? 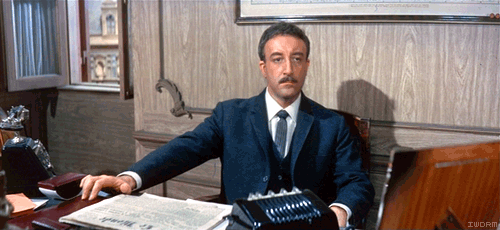 ] <p> </p> .bottom[ If you have any questions for about the content presented herein, please feel free to [submit them to me](https://docs.google.com/forms/d/e/1FAIpQLScrAGM5Zl8vZTPqV8DVSnSrf_5enypyp0717jG4PZiTlVHDjQ/viewform?usp=sf_link) and I'll get back to you as soon as possible.]